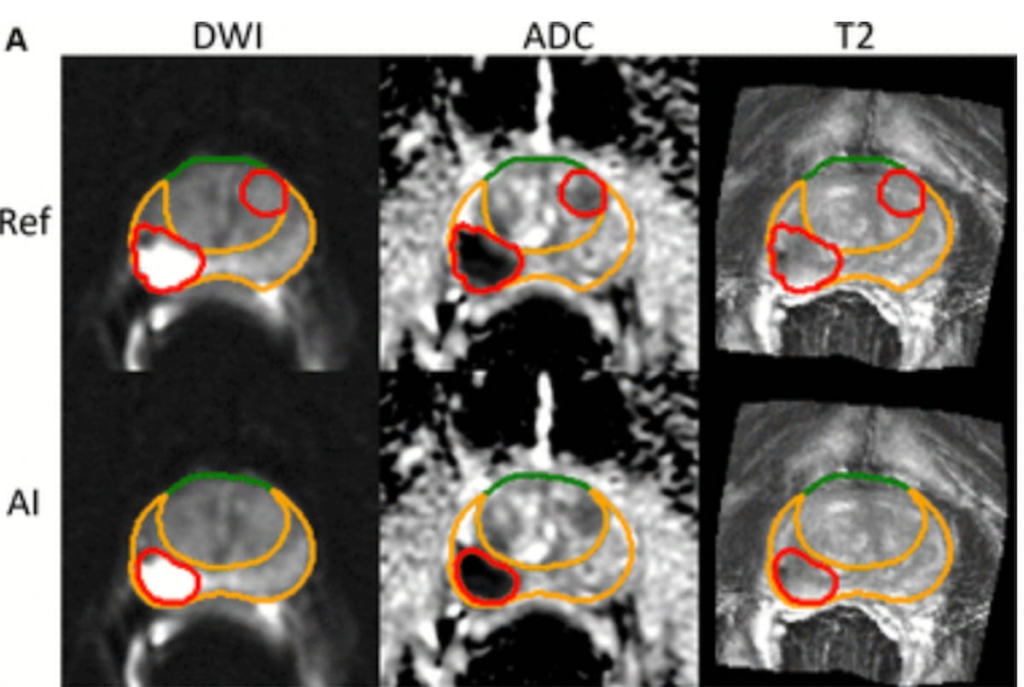
Recent developments in the use of artificial intelligence (AI) for medical imaging are showing promising applications, particularly in the prediction of metastasis in prostate cancer (PCa) patients. A recent study published in the journal Radiology highlights how AI segmentation using multiparametric magnetic resonance imaging (mpMRI) can provide valuable insights into total intraprostatic tumor volume, which is crucial for assessing the likelihood of metastasis among individuals treated for localized prostate cancer.
The study, which was retrospective in nature, involved the crafting and training of an AI-driven tumor segmentation algorithm. The algorithm was tasked with identifying lesions categorized within the Prostate Imaging Reporting and Data System (PI-RADS) scores of 3 to 5. Researchers aimed to compare the AI-generated total tumor volume (VAI) against the traditional National Comprehensive Cancer Network (NCCN) risk classification, analyzing data from 732 patients who underwent radical prostatectomy (RP) or radiotherapy (RT).
The findings revealed that the AI approach significantly outperformed the NCCN risk classification in predicting future metastases. In patients treated with RT, the AI model achieved an 84% score on the area under the receiver operating characteristic curve (AUC) for predicting seven-year metastases. This was higher than the 74% AUC derived from the NCCN classification. For those who underwent RP, the AI model scored an 89% AUC in predicting metastasis within five years, surpassing the NCCN’s 79%.
While the AI system successfully pinpointed a PI-RADS 5 lesion in one area, it missed a PI-RADS 4 lesion elsewhere in MRI scans of a 70-year-old prostate cancer patient. Such findings underscore both the potential and the limitations of AI tools.
According to study lead author Dr. David D. Yang and his team from Brigham and Women’s Hospital and the Dana Farber Cancer Institute in Boston, the predictive power of the AI model is partially due to its consistent measurement of the total burden of significant intraprostatic tumors. Conventional MRI metrics like tumor size and PI-RADS scores, while useful, often suffer from variability due to anatomical changes, imaging inconsistencies, and other factors.
AI models like VAI promote higher reproducibility and are less susceptible to the variations that typically arise from differing biopsy techniques and clinician methodologies. Furthermore, since the AI systematically analyzes the entirety of the prostate, it potentially reduces the chance of under-sampling aggressive disease types, which can sometimes occur in genomic or pathological evaluations that require sample retrievals.
With these insights, AI-assisted tumor volume measurement could potentially influence treatment decisions. Identifying high-risk patients more accurately allows clinicians to consider upfront radiotherapy over surgery, especially in patients with aggressive disease profiles who may benefit more from immediate intervention.
For patients facing unfavorable-risk disease, where there is a high probability of encountering clinically significant adverse outcomes post-prostatectomy, upfront radiotherapy might be the more beneficial choice. This shift in approach highlights the growing role of AI in therapeutic decision-making processes, aiming to enhance prognosis and treatment efficacy.
The study also acknowledged certain limitations, notably its retrospective, single-center design, and the fact that its cohort had PCa diagnoses made prior to the prevalent use of advanced imaging techniques like MRI and ultrasound fusion biopsies. Consequently, the adaptability of the AI algorithm across different MRI systems and settings remains uncertain.
This research underscores the potential for AI in reshaping medical imaging and treatment strategies in oncology, providing a glimpse of a future where more personalized, precise, and efficient cancer care could be realized.