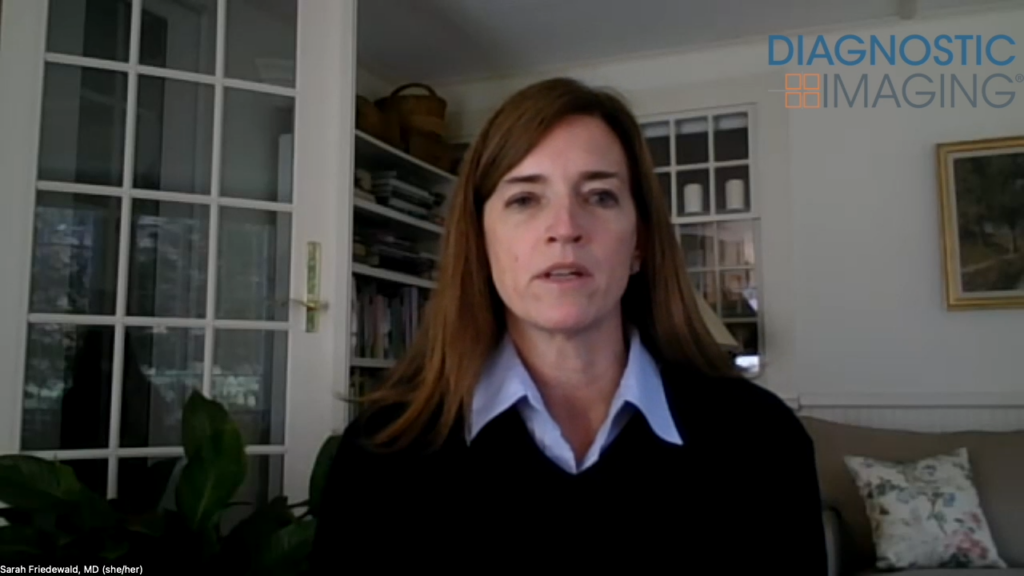
The integration of artificial intelligence (AI) in radiology has held promise for advancements in medical imaging, yet it has also faced the obstacle of insufficiently diverse test sets during algorithm development. This lack of diversity has hampered the ability to ensure equal performance across different racial and ethnic groups. However, recent research has brought hopeful news, particularly with digital breast tomosynthesis (DBT), a mammography technique offering 3D breast imaging. A new AI software named Genius AI Detection 2.0 has demonstrated a promising capability in maintaining consistent diagnostic sensitivity for breast cancer detection across varied demographic groups.
This research was showcased at the Radiological Society of North America (RSNA) conference. The study involved over 7,500 women who underwent DBT, with the cohort comprising 5,395 White women, 956 Black women, 774 Hispanic women, and 279 Asian women. Overall, the AI software achieved a sensitivity rate of 90.1% in detecting breast cancer. Remarkably, the sensitivity was nearly uniform across the racial groups: 90.3% for White women, 88.6% for Black women, 90.1% for Hispanic women, and 91.5% for Asian women.
Dr. Sarah Friedewald, the lead author of the study and vice chair for women’s imaging at Northwestern Medicine Feinberg School of Medicine, expressed her satisfaction with the results. She highlighted the equal performance of the AI across various populations, noting a slight edge in cancer detection for Asian women. In her view, this consistency indicates that AI can be effectively harnessed to balance disparities in breast cancer detection and improve care for all patients, provided there is ongoing monitoring and adaptation of the AI’s performance in diverse settings.
Dr. Friedewald emphasized the potential for AI to play a crucial role in patient triage, suggesting that these tools could ensure that individuals who need further imaging are identified and managed correctly. Real-world application and continuous evaluation remain vital to realize these benefits fully, but the current findings offer a strong foundation for moving towards more equitable healthcare.
This study adds to a growing body of research illustrating the potential of AI to transform traditional medical screening methods, extending their reach and efficacy. While previous AI applications in breast cancer screening have shown promise, this particular software stands out by demonstrating reliable performance across different demographic groups, which is a critical step toward reducing healthcare disparities.
Building on the promise of AI in mammography, other studies have shown enhanced cancer detection rates when adjunctive AI screening was incorporated, and updated AI platforms for digital breast tomosynthesis have been cleared by the FDA. These advancements collectively highlight the transformative potential of AI in healthcare, particularly in reducing racial and ethnic disparities in cancer detection.
For further insights into these developments and more expert opinions, supplementary materials and video interviews with key researchers, including Dr. Friedewald, are available. These resources offer a comprehensive view of how AI is poised to enhance breast cancer screening and ensure that technological advancements benefit everyone equally, regardless of their race or ethnicity.
In conclusion, while the path forward with AI in radiological imaging is promising, continuous research and careful application remain essential. The goal is an inclusive healthcare future where every patient receives accurate and prompt detection of diseases like breast cancer, leveraging the full capabilities of AI technology.