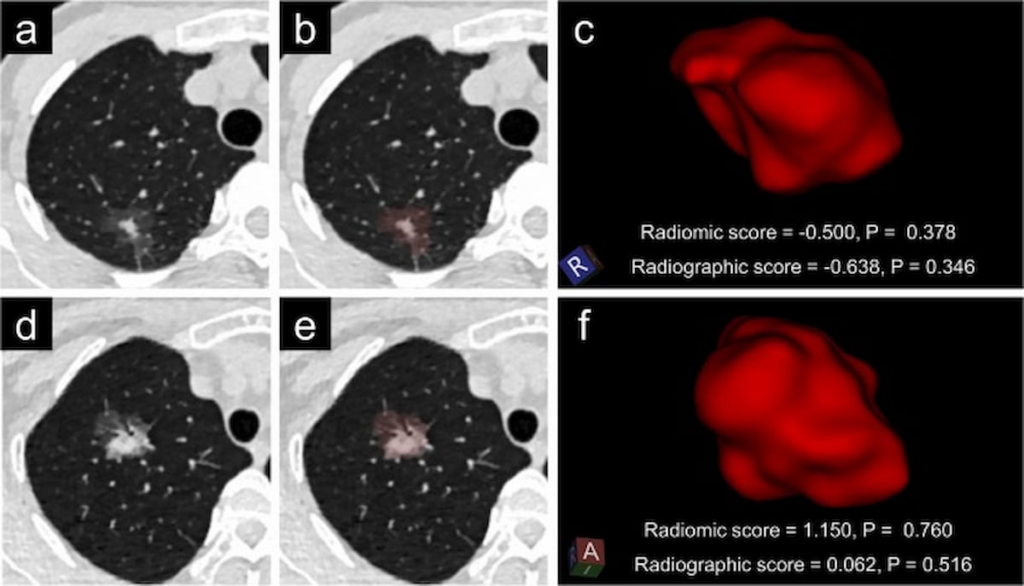
Low-Dose Computed Tomography Offers Enhanced Predictive Capabilities for Lung Adenocarcinoma
Research has long been underway to develop more effective methods for diagnosing high-risk lung adenocarcinoma (LUAD) patients. Recent findings suggest that a novel radiomic model based on low-dose computed tomography (LDCT) may hold the key to improved prognostic capabilities.
To determine the efficacy of this innovative model, researchers conducted a multicenter retrospective study involving 658 patients diagnosed with LUAD. The retrospective analysis evaluated the diagnosis of both radiomic and radiographic models and compared their performance in predicting high-risk lung adenocarcinoma.
In contrast to the radiographic model, the radiomic model demonstrated notable enhancements in accuracy, according to the findings. The radiomic model boasted a much higher area under the curve (AUC) for predicting high-risk LUAD with a rate of 93.8 percent compared to 88 percent for the radiographic model.
Another notable difference was observed in the sensitivity of the models. The radiomic model showed a higher sensitivity with a rate of 79.1 percent, compared to 76.7 percent for the radiographic model. Moreover, the radiomic model presented notably higher specificity and positive predictive value rates, with 89.2 percent and 82.9 percent respectively, as opposed to 78.5 percent and 70.2 percent for the radiographic model.
These impressive results have significant implications for personalized clinical decision-making processes during lung cancer screening. Additionally, they imply that radiomic models like the one presented in the study could be a non-invasive biomarker for the identification of high-risk LUADs.
Researchers found that the radiomic model was primarily based on texture features of the images, including GLCM_Correlation, a feature that gauges the linear dependency of gray-level values on each other in respective voxels. Astonishingly, this feature alone demonstrated a 2.7-fold higher risk of developing high-risk lung adenocarcinoma.
Other radiomic features like GLDM_LargeDependenceHighGrayLevelEmphasis and GLSZ_SmallAreaEmphasis were also found to be associated with LUAD occurrence, indicating tumor heterogeneity that could not be described using traditional radiographic features.
According to Jieke Lu, a researcher affiliated with the Department of Radiology with the Sichuan Clinical Research Center for Cancer, the radiomic score, based on LDCT data, might be a useful marker to guide clinical decision-making during lung screening.
The study’s limitations were noted by the researchers, including possible patient selection bias and the use of a relatively small sample size to develop and validate the radiomic model. To address these concerns, further research is required, with a particular emphasis on prospective studies that can be used to determine the applicability of the radiomics model to both standard-dose and LDCT data, as well as provide greater insight on different types of scanners and acquisition criteria.