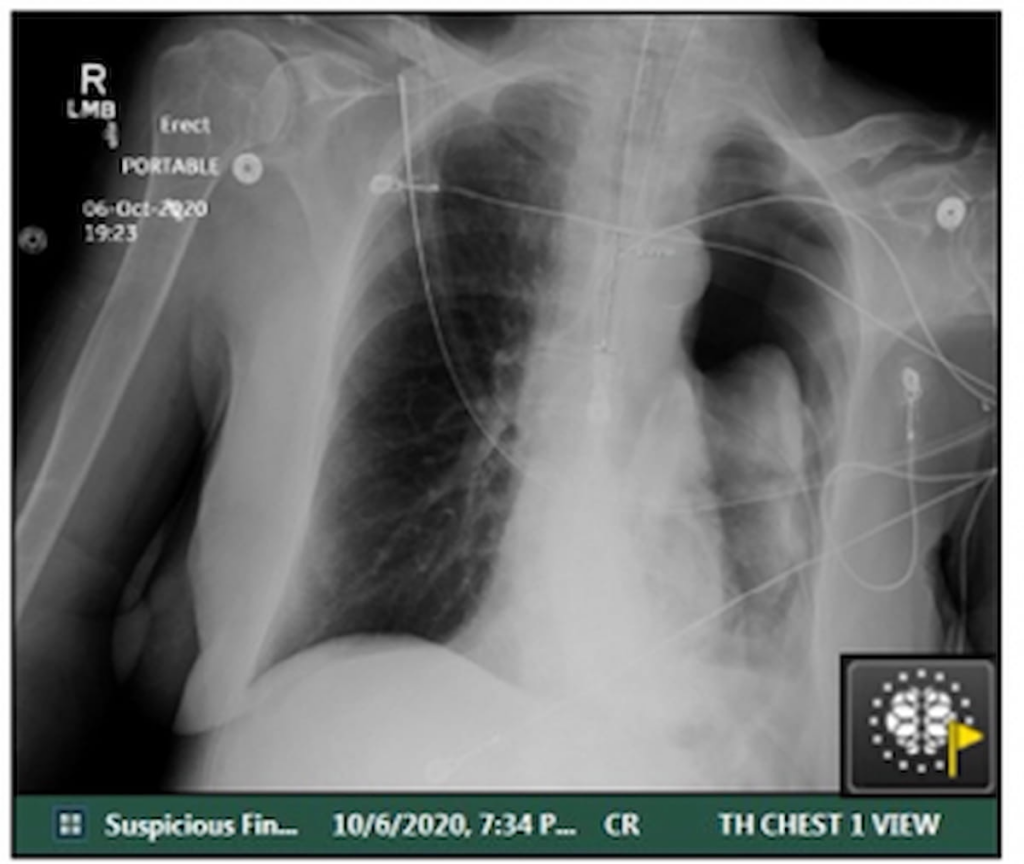
A recent retrospective study published in Academic Radiology reveals promising findings regarding an artificial intelligence (AI) algorithm’s performance in detecting pneumothorax—a potentially deadly condition where air enters the pleural space, causing lung collapse. The study highlights the AI’s efficiency, showing an 86-minute reduction in median reporting time for clinically actionable cases.
Researchers compared an AI-based pneumothorax detection tool (Critical Care Suite 1.0 by GE HealthCare) with traditional PACS triage systems on 12,728 chest X-rays and against standard practice on 14,669 X-rays. The AI system demonstrated a 78 percent area under the curve (AUC) with 97 percent sensitivity and 60 percent specificity. Notably, for moderate to large pneumothorax cases, the AI showed impressive results, with a 93 percent AUC and 89 percent sensitivity, effectively matching the sensitivity range of radiologists, typically around 85 percent.
In one illustrative case, AI quickly flagged a suspected pneumothorax on a post-operative X-ray for a COVID-19 patient with lung cancer. The critical findings were communicated to the attending physician within just 21 minutes after the scan, underscoring the AI’s potential to expedite urgent clinical decisions.
One of the study’s authors, Dr. Amit Gupta from University Hospitals Cleveland Medical Center, emphasized that the AI’s accuracy is commendable, especially when benchmarked against real-world clinical data. The research also highlighted significant improvements in workflow, facilitated by the AI tool, particularly during on-call hours. For routine pneumothorax cases, AI usage resulted in a 57.1 percent decrease in median reporting time, slashing it from 345 minutes in the control group to just 148 minutes.
The study identifies three key advantages of using AI in this context. Firstly, the algorithm exhibits robust detection capabilities, particularly for moderate and large pneumothorax, with a high specificity of 97 percent. Secondly, the AI brings significant enhancements to workflow efficiency; median reporting times for all positive pneumothorax cases dropped by 46.2 percent, highlighting improvements across different case priorities. Lastly, the AI’s triage effectiveness appears to extend beyond existing STAT priority protocols, showing promise in high-volume settings.
Further, the AI tool contributed to a 29.3 percent reduction in reporting times for STAT priority cases (from 92 to 65 minutes) and a 46.2 percent reduction across all cases with positive findings (from 186 to 100 minutes). According to Gupta and his team, these improvements suggest that AI integration in triaging pneumothorax can enhance workflows beyond traditional prioritization methods like STAT ordering.
Despite these promising results, the authors acknowledged some limitations. The primary AI-enabled scanner was primarily used in the ICU, while the control group sometimes utilized non-AI scanners in various settings including emergency departments. This discrepancy could have contributed to the uneven distribution of confirmed pneumothorax cases between the control group and the AI cohort.
In conclusion, the implementation of AI in detecting and triaging pneumothorax shows great potential for improving diagnostic accuracy and streamlining workflow in busy clinical environments. Although the technology proves to be a significant aid, further studies are required to address the limitations and ensure its applicability across different settings.