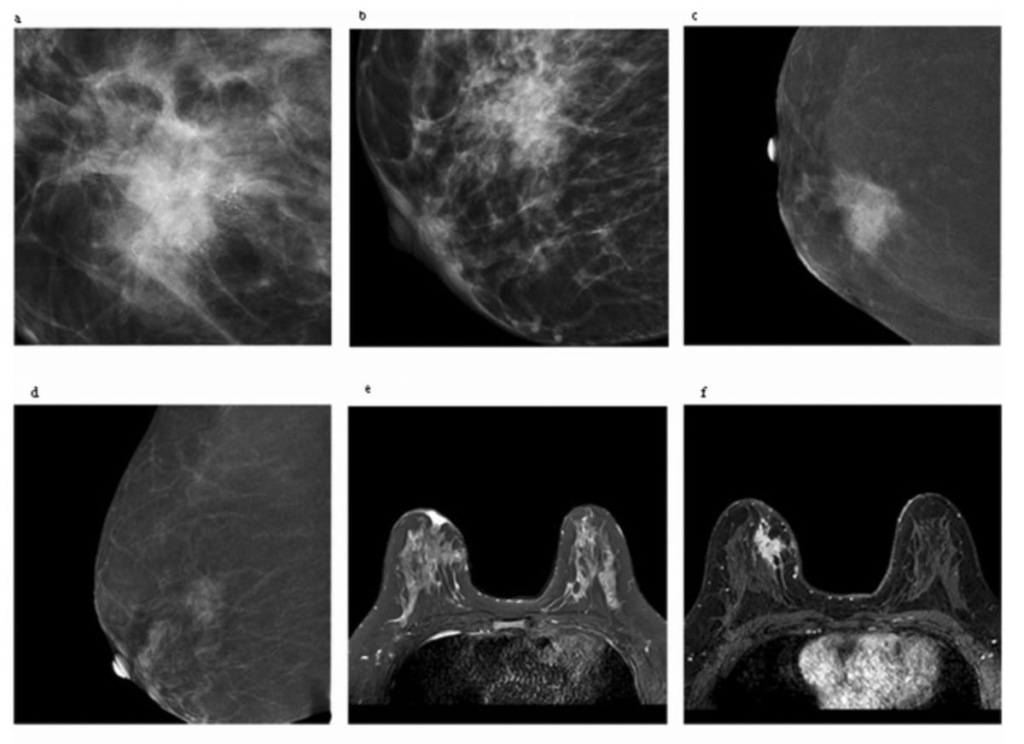
Recent research highlights the potential of the machine learning-derived Kaiser score (KS) in enhancing the detection of breast cancer in contrast-enhanced mammography (CEM) and breast magnetic resonance imaging (MRI). According to findings published in Academic Radiology, the KS may provide improved performance compared to the established Breast Imaging Reporting and Data System (BI-RADS) when assessing breast lesions in women.
The study, which involved a total of 275 patients with documented breast-enhanced lesions, aimed to evaluate the efficacy of KS against BI-RADS. The patients had a mean age of approximately 49 years and accounted for 281 lesions. The researchers conducted additional analyses on a subgroup of 149 women who underwent both CEM and MRI, totaling 151 lesions. The KS incorporated various features such as the lesion’s margin, root sign, internal enhancement pattern (IEP), the type of time-signal intensity curve (TIC), and edema observable on MRI.
The results were noteworthy: for CEM, the KS achieved an impressive area under the curve (AUC) of 89.7%, significantly outperforming the BI-RADS CEM assessment, which stood at 69.1%. Although BI-RADS showed superior sensitivity (98.8% compared to 89.2%) and a higher negative predictive value (NPV) of 95.74% versus 86.84%, it also exhibited more than a 30% lower specificity rate (39.47% vs. 69.72%) and a diminished positive predictive value (PPV) of 70.51% compared to the KS at 74.25%.
In a closer examination of women who had both CEM and MRI, the performance of KS-MRI held up well, achieving an AUC of 87.6% relative to 74% for BI-RADS MRI. BI-RADS MRI presented higher sensitivity levels (96.55% versus 91.23%) and NPV (81.82% compared to 71.43%), but KS-MRI demonstrated a notable advantage in specificity (67.57% vs. 51.43%) and a higher PPV of 89.66% compared to 86.82% with BI-RADS.
Dr. Bei Hua, affiliated with the Department of Radiology and Nuclear Medicine at the First Hospital of Hebei Medical University, emphasized that although BI-RADS provides a framework of descriptors and assessment categories, it lacks definitive guidelines for managing lesions exhibiting particular features. The introduction of the KS could address this gap, enhancing the overall performance of breast imaging diagnoses.
Further observations in the study revealed that the AUCs for KS-CEM and KS-MRI were comparable, indicating that both imaging modalities have similar detection capabilities. The KS demonstrated an 83.19% accuracy rate in identifying irregular lesion margins associated with malignancy, closely following the 89.16% accuracy rate for KS-MRI. The researchers noted that CEM could potentially improve breast cancer detection rates while also reducing the chances of misdiagnosis, rivaling the diagnostic efficiency of breast MRI.
Key takeaways from this research include the following:
-
The machine learning-based Kaiser score exhibited a higher specificity for CEM compared to BI-RADS, with specificity rates of 69.72% versus 39.47%.
-
For KS-MRI, although BI-RADS MRI showed better sensitivity and NPV, KS-MRI outperformed it significantly in terms of specificity (67.57% vs. 51.43%) and offered a higher PPV, suggesting it could minimize false positives through more accurate diagnoses.
- The AUC values were closely matched for both KS-CEM and KS-MRI, at 87.8% and 87.6% respectively, underscoring that CEM can deliver diagnostic accuracy that approaches that of MRI.
The study acknowledged several limitations, including its single-facility focus and a relatively small cohort size, which may impact the generalizability of findings. Moreover, certain lesions, particularly non-enhancing or non-mass enhance lesions, were excluded, which may have restricted the full application of TIC and internal enhancement patterns in the analysis.
In conclusion, the adoption of the Kaiser score has the potential to significantly enhance breast cancer detection in women, particularly when assessed through contrast-enhanced imaging techniques. This advancement could lead to more accurate diagnosis, better management of breast lesions, and ultimately, improved patient outcomes.